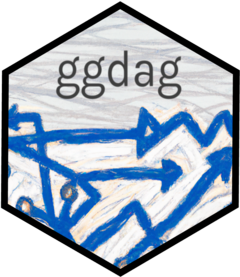
Query and Test Conditional Independence in a DAG
Source:R/implied_conditional_independence.R
query_conditional_independence.Rd
query_conditional_independence()
queries conditional independencies implied
by a given DAG. These serve as potential robustness checks for your DAG.
test_conditional_independence()
runs the tests of independence implied by
the DAG on a given dataset. ggdag_conditional_independence()
plots the
results as a forest plot.
Usage
query_conditional_independence(
.tdy_dag,
type = "missing.edge",
max.results = Inf
)
test_conditional_independence(
.tdy_dag,
data = NULL,
type = c("cis", "cis.loess", "cis.chisq", "cis.pillai", "tetrads", "tetrads.within",
"tetrads.between", "tetrads.epistemic"),
tests = NULL,
sample.cov = NULL,
sample.nobs = NULL,
conf.level = 0.95,
R = NULL,
max.conditioning.variables = NULL,
abbreviate.names = FALSE,
tol = NULL,
loess.pars = NULL
)
ggdag_conditional_independence(
.test_result,
vline_linewidth = 0.8,
vline_color = "grey70",
pointrange_fatten = 3
)
Arguments
- .tdy_dag
A tidy DAG object.
- type
can be one of "missing.edge", "basis.set", or "all.pairs". With the first, one or more minimal testable implication (with the smallest possible conditioning set) is returned per missing edge of the graph. With "basis.set", one testable implication is returned per vertex of the graph that has non-descendants other than its parents. Basis sets can be smaller, but they involve higher-dimensional independencies, whereas missing edge sets involve only independencies between two variables at a time. With "all.pairs", the function will return a list of all implied conditional independencies between two variables at a time. Beware, because this can be a very long list and it may not be feasible to compute this except for small graphs.
- max.results
integer. The listing of conditional independencies is stopped once this many results have been found. Use
Inf
to generate them all. This applies only whentype="missing.edge"
ortype="all"
.- data
matrix or data frame containing the data.
- tests
list of the precise tests to perform. If not given, the list of tests is automatically derived from the input graph. Can be used to restrict testing to only a certain subset of tests (for instance, to test only those conditional independencies for which the conditioning set is of a reasonably low dimension, such as shown in the example).
- sample.cov
the sample covariance matrix; ignored if
data
is supplied. Eitherdata
orsample.cov
andsample.nobs
must be supplied.- sample.nobs
number of observations; ignored if
data
is supplied.- conf.level
determines the size of confidence intervals for test statistics.
- R
how many bootstrap replicates for estimating confidence intervals. If
NULL
, then confidence intervals are based on normal approximation. For tetrads, the normal approximation is only valid in large samples even if the data are normally distributed.- max.conditioning.variables
for conditional independence testing, this parameter can be used to perform only those tests where the number of conditioning variables does not exceed the given value. High-dimensional conditional independence tests can be very unreliable.
- abbreviate.names
logical. Whether to abbreviate variable names (these are used as row names in the returned data frame).
- tol
bound value for tolerated deviation from local test value. By default, we perform a two-sided test of the hypothesis theta=0. If this parameter is given, the test changes to abs(theta)=tol versus abs(theta)>tol.
- loess.pars
list of parameter to be passed on to
loess
(fortype="cis.loess"
), for example the smoothing range.ciTest(X,Y,Z,data)
is a convenience function to test a single conditional independence independently of a DAG.- .test_result
A data frame containing the results of conditional independence tests created by
test_conditional_independence()
.- vline_linewidth
Line width for the vertical line indicating no effect.
- vline_color
Color of the vertical line.
- pointrange_fatten
Factor to fatten the point range.